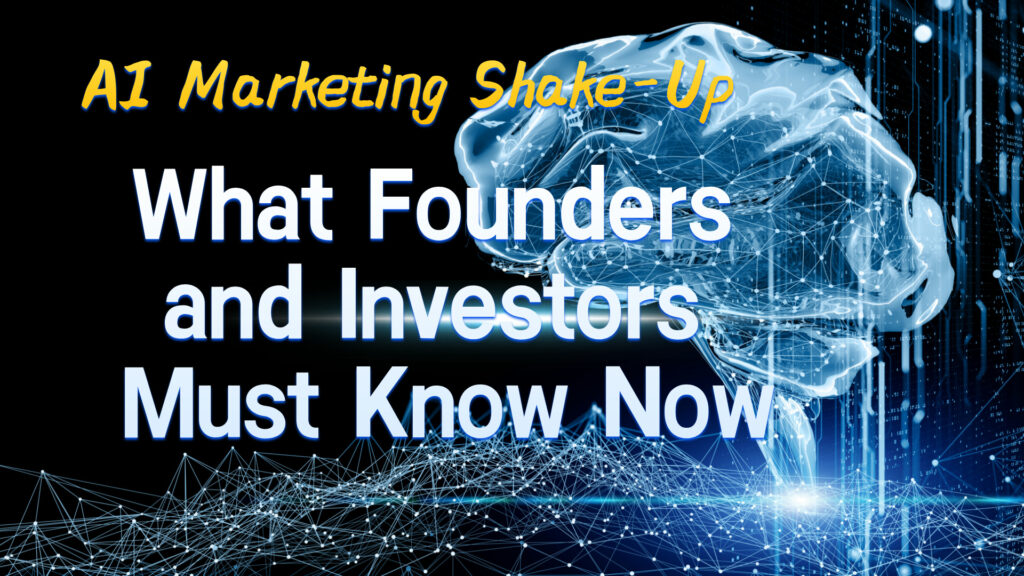
With a core philosophy rooted in long-termism and building an integrated industrial ecosystem, Sequoia Capital recently hosted its third annual AI Summit. The event brought together 150 of the world’s leading AI founders and conveyed three powerful signals.
Three Core Signals for the AI Industry in the Next Decade
1. AI Business Models: The Shift From “Tool-Oriented” to “Outcome-Oriented”
This transformation reflects a deeper move toward closing the loop in AI-driven business models, where technology translates into measurable business outcomes.
Over the past decade, enterprise software has delivered value primarily by improving operational efficiency. Companies paid for SaaS tools that automated workflows — essentially, “software as a tool.” But AI is now breaking through that model and shaping a new paradigm:
Software as a Tool → Software as a Co-worker → Software as an Outcome
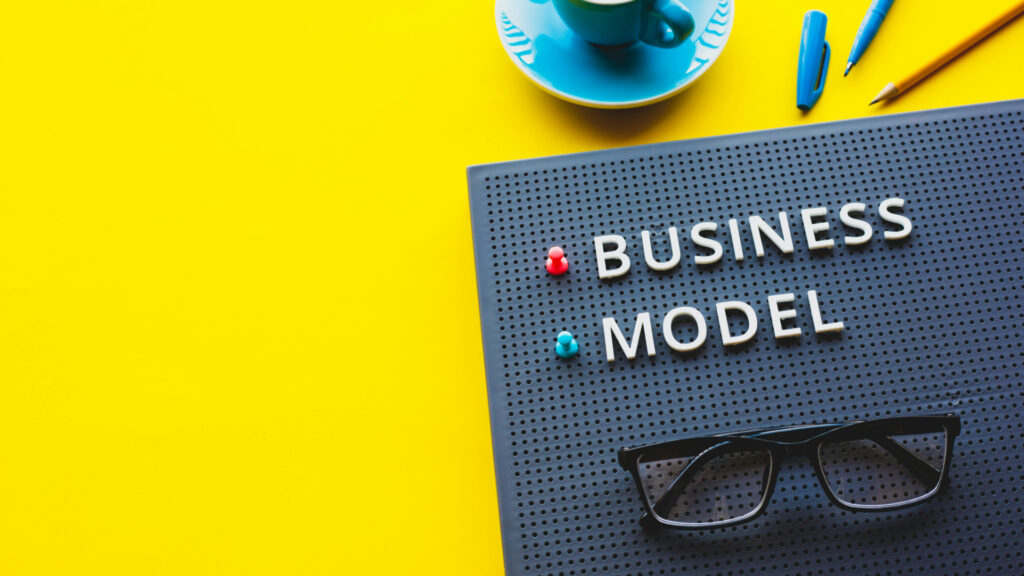
In this new framework, users are no longer paying for the AI model’s capabilities alone — they’re paying for its ability to solve real-world problems and deliver results.
Take a legal AI startup as an example. It has evolved from offering simple API-based services to delivering complete legal documents, shifting its value proposition from tool to outcome — and in doing so, creating a self-contained value loop.
2. Competition for AI “Operating System” — Level Entry Points
At the core of this race is the strategic goal of building platform-level moats through enhanced user stickiness. This stickiness is fundamentally driven by two key capabilities: memory (the accumulation of historical user-AI interactions) and execution (the AI’s ability to efficiently orchestrate tools and complete tasks).
Memory: Evolving from a “Tool” to a “Digital Companion”
Long-term memory enables AI systems to deeply learn a user’s habits, preferences, and contextual needs, unlocking truly personalized, one-to-one experiences at scale.
This memory capability shifts AI from being a passive command responder to an active need interpreter, evolving into a digital companion similar to a human assistant. For example, in healthcare, an AI system that incorporates a patient’s medical history, genetic profile, and medication records can dynamically adjust treatment recommendations — moving beyond one-size-fits-all suggestions.
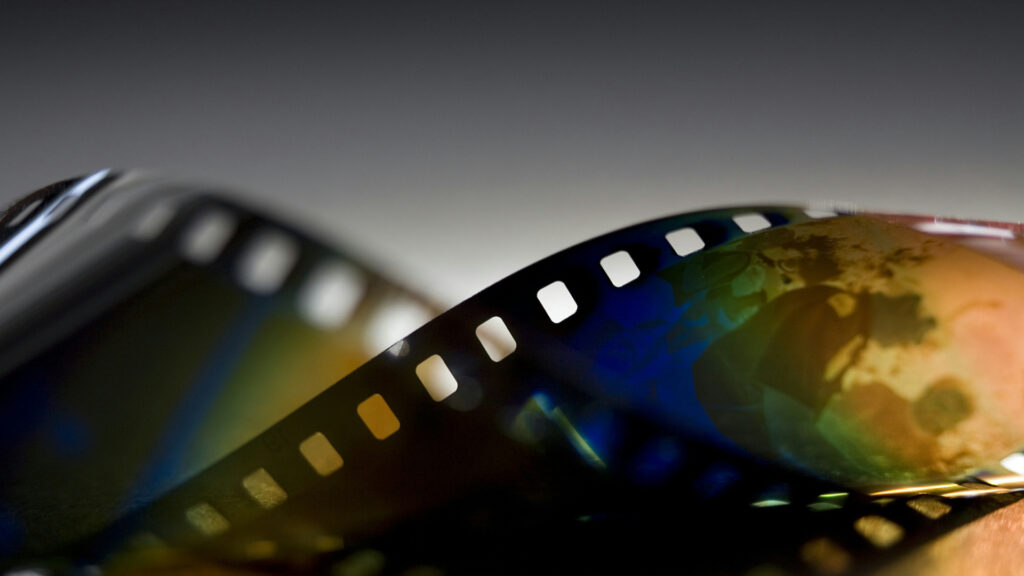
Execution: Evolving from “Capability Showcase” to “Task Completion Loop”
An AI’s ability to efficiently call external tools (e.g., APIs, databases, hardware devices) determines whether it can execute end-to-end workflows in complex real-world scenarios. Execution efficiency is a critical adoption barrier for enterprise AI.
For instance, a legal AI tool that can autonomously pull from contract templates, search relevant case law, and coordinate with a legal team far outperforms traditional methods reliant on manual operations.
When deep memory and high execution efficiency come together, they form an irreversible user dependency, building a powerful moat for the AI platform. This is the foundation for the next generation of AI-native operating systems.
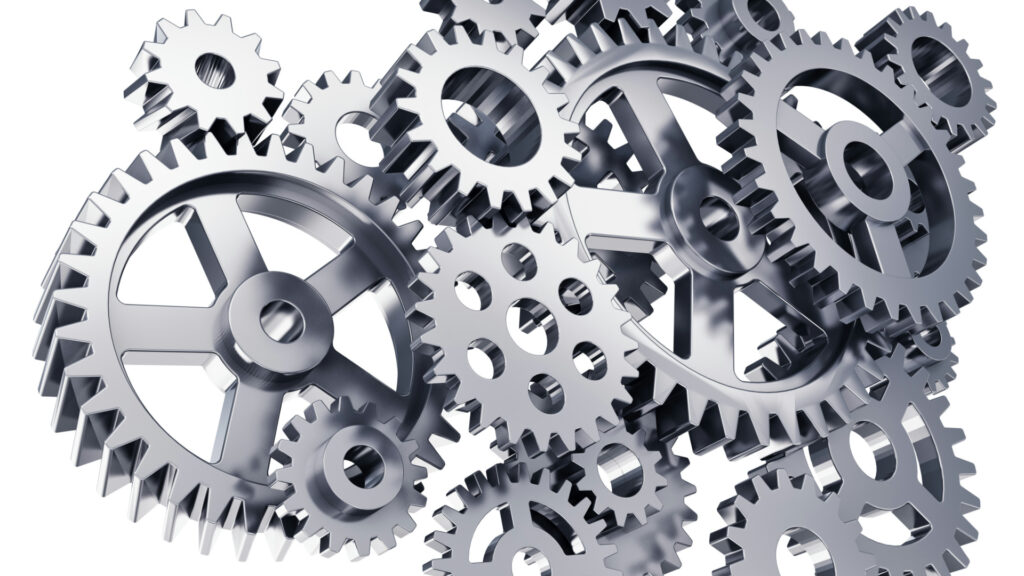
3. The Rise of the Agent Economy: From Tools to Autonomous Economic Actors
Artificial intelligence is rapidly evolving from a passive tool into an autonomous economic participant, giving rise to the “agent economy.” To realize this vision, three foundational pillars must be established:
1). Persistent Identity: Building the “Digital Persona” of Agents
Each agent must possess a unique and verifiable identity to establish its legitimacy within an economic system. For example, China’s dual-track digital identity framework — “Net ID + Net Credential” — offers a policy and technical foundation for authenticating AI agents.
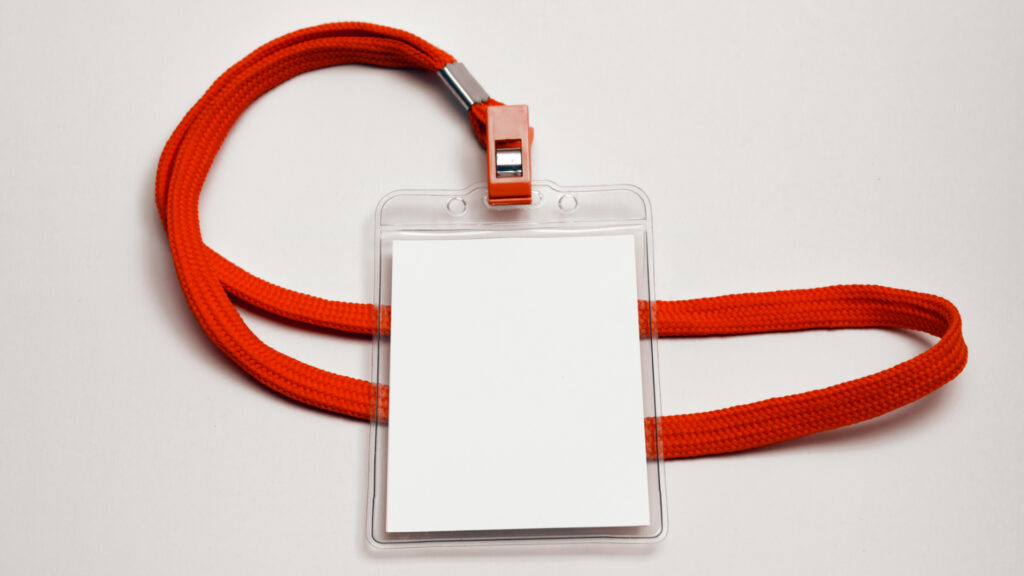
2). Action Capability: Bridging the Digital and Physical Worlds
Agents must be able to orchestrate both physical tools (such as robots and IoT devices) and digital resources (like APIs and databases) to complete closed-loop tasks. Key challenges include permission management, real-time responsiveness, and robust fault tolerance (e.g., automatic retries or fallback to human intervention in case of failure).
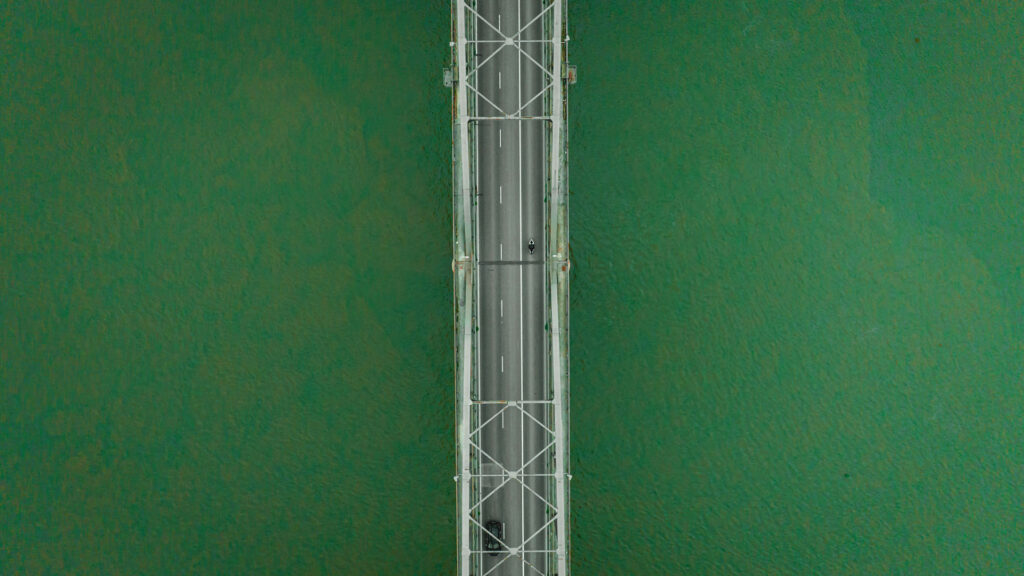
3). Trustworthy Collaboration: A Trust Infrastructure for Inter-Agent Cooperation
Collaboration between agents requires a trustworthy infrastructure that ensures transparency, traceability, and accountability. A central question: How do we assign responsibility among autonomous agents? The LOKA Protocol, developed by Carnegie Mellon University, proposes a layered framework (Identity Layer + Communication Layer + Ethics Layer) that supports decentralized identity and ethical decision-making for agents.
Additionally, how can we balance data privacy and information sharing in a world of interacting agents? This tension will define the future landscape of agent-based collaboration.
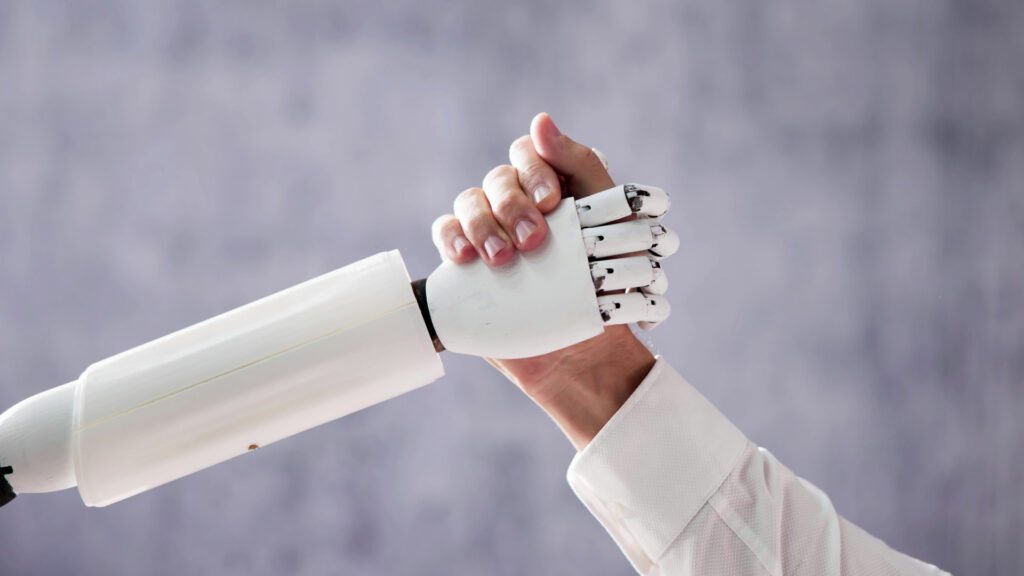
The Essence of Competitive Advantage: A Trinity of Data – Use Case – Ecosystem
In summary, the evolution of the AI industry presents both strategic inflection points and evaluation criteria for investors and startup founders: the ability to build sustainable competitive moats while closing the revenue loop.
According to Sequoia Capital, long-term defensibility in AI companies hinges on three pillars:
- Data Flywheel:
The efficiency of feedback loops where user interaction data continuously improves model performance. - Scenario Depth:
Irreplaceable value in solving domain-specific, complex problems—especially in verticals like healthcare and law. - Ecosystem Synergy:
Building a collaborative network across stakeholders through platform-level products or standardized protocols.
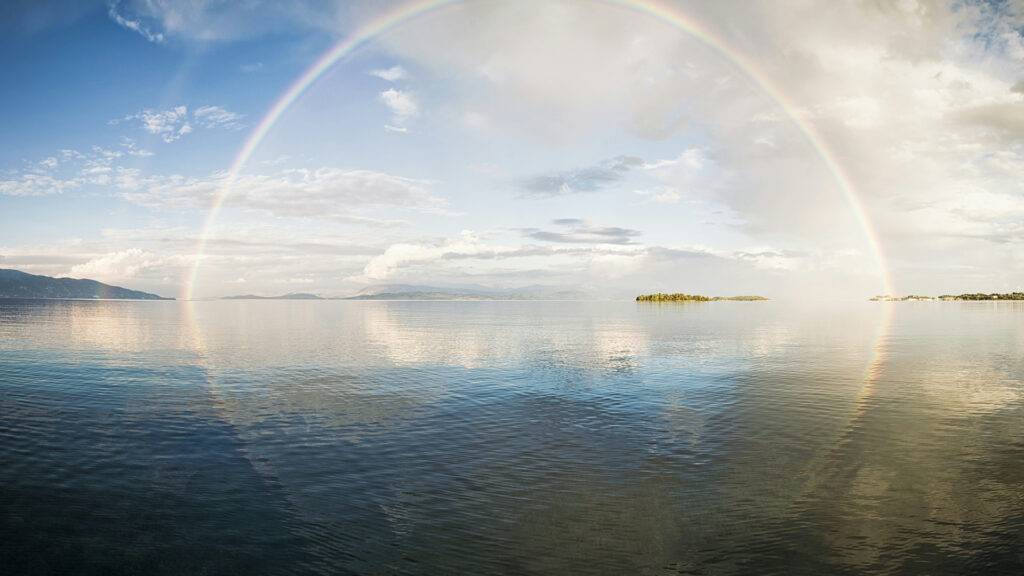
Sequoia predicts that by 2026, vertical AI agents and hybrid governance frameworks will drive the emergence of trillion-dollar markets. Conversely, companies that fail to evolve from tool → collaborator → outcome will be left behind.